GAIN a competitive edge for your sorting operation
We'll tell you how!
GAIN: A giant breakthrough in sorting accuracy
As a pioneering add-on technology for the AUTOSORT®, GAIN makes it possible to sort objects which could previously not been separated based on their form and texture. Whereas previously only available for the purification of PE streams, GAIN now delivers exceptional results in wood sorting too, making you reach higher purity and productivity levels.
Smarter sorting solutions, today and tomorrow
Deep learning is a technology that analyzes images and data to enable the sorting unit to perform tasks more effectively over time – and proves very promising for waste management and recycling. When being trained and exposed to thousands of images, GAIN draws connection and continuously learns how to differentiate recyclable from non-recyclable waste items, including previously difficult to sort materials.
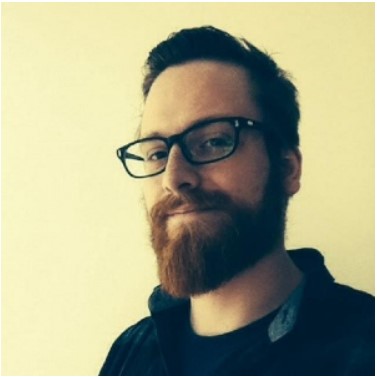
"At TOMRA, we’ve used Artificial Intelligence for decades, and we’re confident that deep learning will continue to add tremendous value for recyclers. That’s why we’re investing heavily in software development to expand our deep learning capabilities across more applications. We want to bring innovative solutions to sorting challenges that cannot be solved with conventional technology"
Sebastian Solbach
Team Leader Application Development - Deep Learning | R&D
Explore our current deep learning-based applications
New: GAIN for wood chips
Pioneering the use of deep learning, GAIN is now available for wood recycling applications. GAIN enables your operation to create clean recycled wood chip fractions and differentiate between non-processed wood (wood A) and processed wood (wood B).
While x-ray transmission (XRT) sorting technology excels at removing impurities like inert materials, metals and glass from wood, GAIN goes the extra mile to classify and purify waste wood by distinguishing between various types of wood-based materials, including: MDF, HDF, WPC and wood composites.
Your benefits:
- Higher yield and purities
- Sorts waste wood categories
- Increased process control
- Robust and flexible sorting system
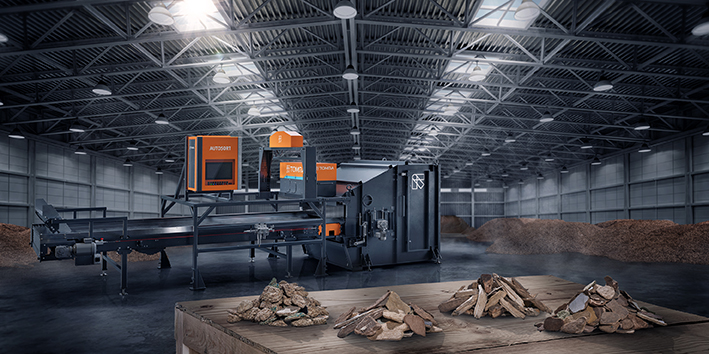
GAIN purifies wood chips by removing impurities such as:
- MDF (medium density fiberboard)
- HDF (high density fiberboard)
- WPC (wood polymer composites)
- OSB (oriented strand board)
- Chipboard
- Plywood

"Our new AUTOSORT® with the GAIN technology makes us the first company in the world to use deep learning to detect and separate different wood types. It’s a groundbreaking innovation that allows recyclers to process high-quality fractions by categories like processed vs non-processed wood.”
Philipp Knopp
Product Manager Recycling
GAIN for silicone cartridges
Used in combination with AUTOSORT, GAIN purifies post-consumer polyethylene (PE) by removing PE-silicone cartridges and non-PE objects. Until now, silicone cartridges were considered difficult to sort and a major contaminant in PE waste streams – but GAIN sets new industry standards in performance and purity levels. Even if the silicon cartridges are bundled, damaged or deformed, GAIN detects and separates the objects from clean PE fractions, giving plastic recyclers a significant competitive advantage.
Your benefits:
- Unrivaled post-consumer PE purity levels
- Great adaptability
- Supports maximum belt speed
Deep Learning explained
Transforming image data into adaptive sorting intelligence
TOMRA’s deep learning system is used for the categorization of an object based on an image. As a subset of AI, deep learning extracts meaningful features from collected data, e.g., images or videos, based on which the solution learns to better understand unique object characteristics and how to classify the objects transported on the conveyor belt and scanned by the sensors.
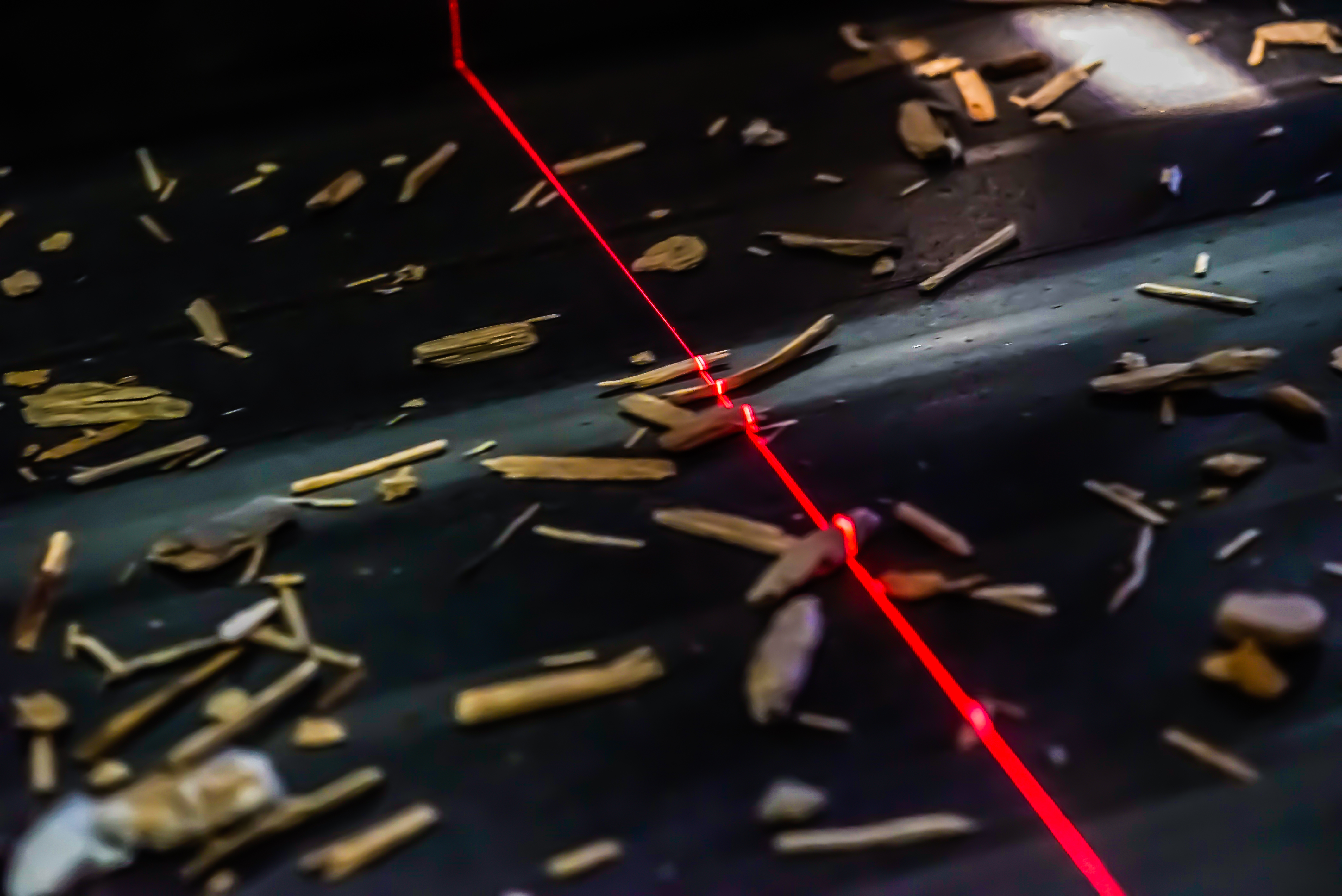
Neural networks connecting the dots
Deep learning technology brings a new dimension of performance, but its success depends on the construction of its neural networks that require extensive labeled data to train the sorting system. TOMRA software engineers train the network with thousands of images that hold a pool of object information. Based on this information, the network recognizes patterns and properties, connects the information pool to the sorting task for highly efficient sorting.
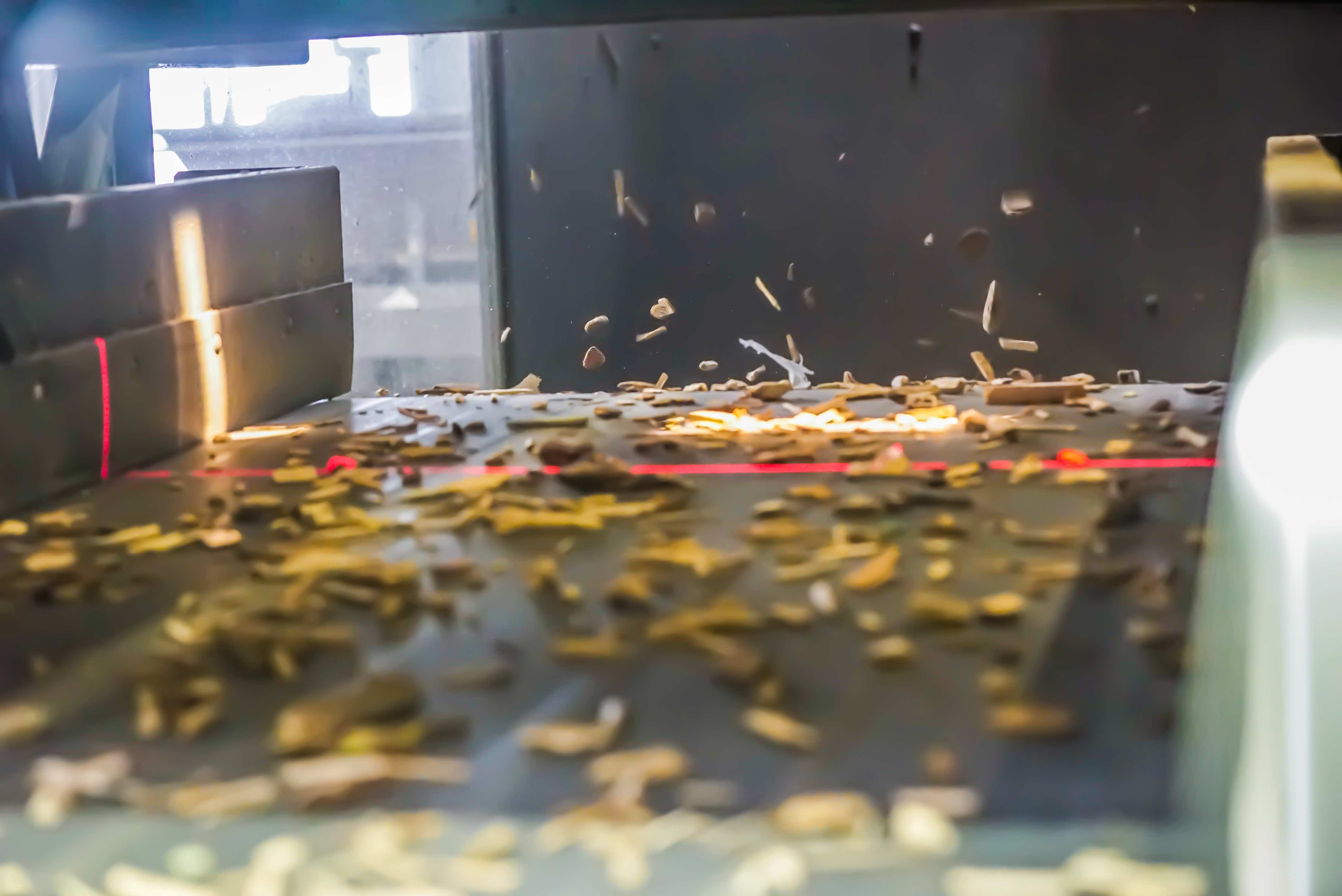